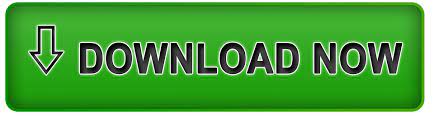

Similarly, if your model has x, z, and xz, both x and z are likely to be highly correlated with their product. If you specify a regression model with both x and x 2, there’s a good chance that those two variables will be highly correlated. The high VIFs are caused by the inclusion of powers or products of other variables. So there’s no problem to be concerned about, and no need to delete one or the other of the two controls.Ģ. But the VIF for the public/private indicator is only 1.04. 9, which corresponds to VIFs of at least 5.26 for each of them. These two variables have a correlation above. Two control variables are average SAT scores and average ACT scores for entering freshmen. colleges, the dependent variable is graduation rate, and the variable of interest is an indicator (dummy) for public vs. Here’s an example from some of my own work: the sample consists of U.S. The coefficients of the variables of interest are not affected, and the performance of the control variables as controls is not impaired. But so long as the collinear variables are only used as control variables, and they are not collinear with your variables of interest, there’s no problem. It increases the standard errors of their coefficients, and it may make those coefficients unstable in several ways. Here’s the thing about multicollinearity: it’s only a problem for the variables that are collinear. The variables with high VIFs are control variables, and the variables of interest do not have high VIFs. Regardless of your criterion for what constitutes a high VIF, there are at least three situations in which a high VIF is not a problem and can be safely ignored:ġ. Personally, I tend to get concerned when a VIF is greater than 2.50, which corresponds to an R 2 of.
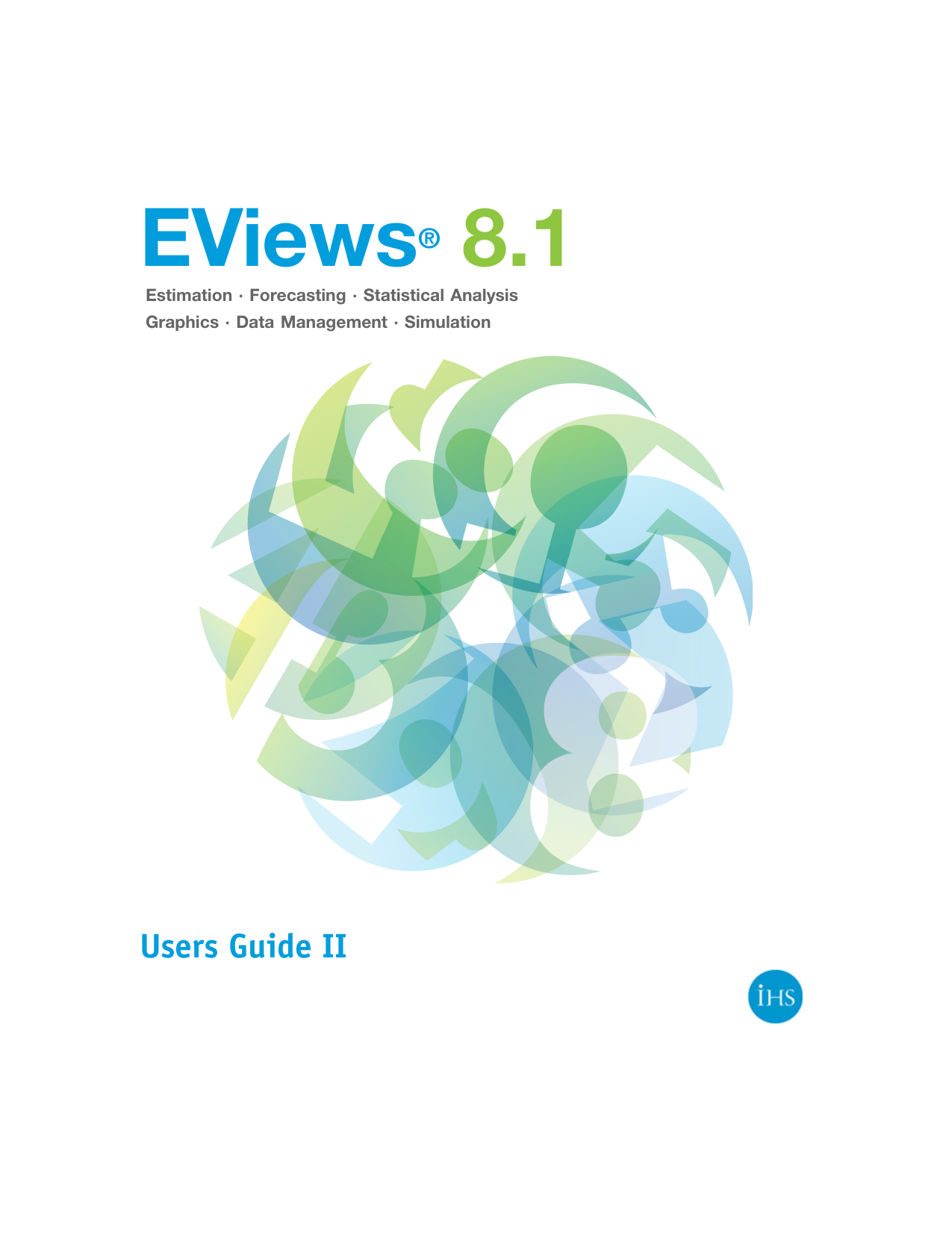
Authorities differ on how high the VIF has to be to constitute a problem. The VIF has a lower bound of 1 but no upper bound. Thus, a VIF of 1.8 tells us that the variance (the square of the standard error) of a particular coefficient is 80% larger than it would be if that predictor was completely uncorrelated with all the other predictors. It’s called the variance inflation factor because it estimates how much the variance of a coefficient is “inflated” because of linear dependence with other predictors. LEARN MORE IN A SEMINAR WITH PAUL ALLISON The VIF may be calculated for each predictor by doing a linear regression of that predictor on all the other predictors, and then obtaining the R 2 from that regression. But many do not realize that there are several situations in which multicollinearity can be safely ignored.īefore examining those situations, let’s first consider the most widely-used diagnostic for multicollinearity, the variance inflation factor (VIF). Most data analysts know that multicollinearity is not a good thing. It occurs when there are high correlations among predictor variables, leading to unreliable and unstable estimates of regression coefficients. Multicollinearity is a common problem when estimating linear or generalized linear models, including logistic regression and Cox regression. When Can You Safely Ignore Multicollinearity? SeptemBy Paul Allison
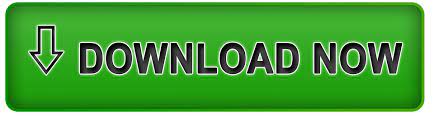